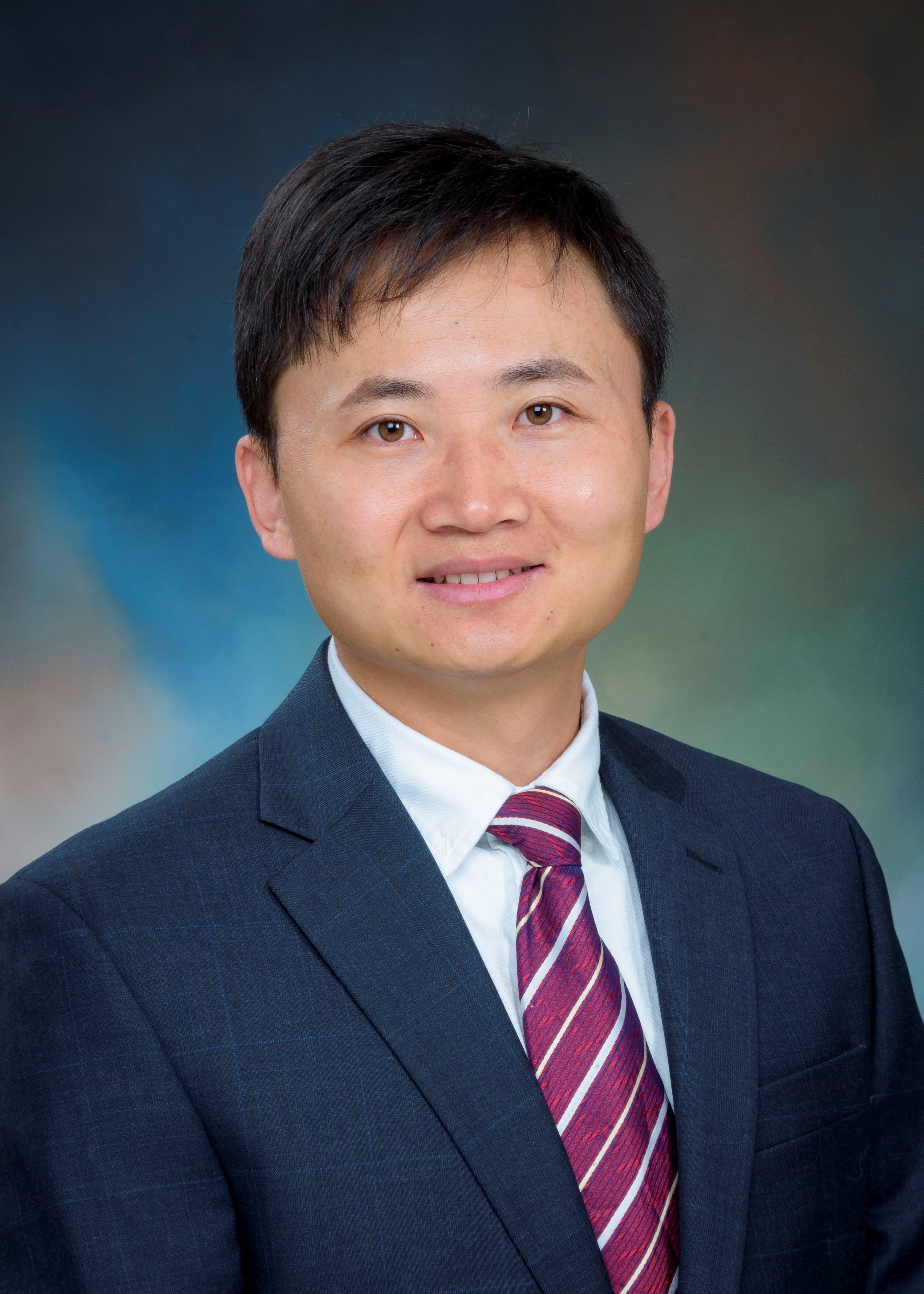
Huiwen Xu
About
I am an aging-focused health services researcher with extensive experience studying long-term care, health policy, and cancer survivorship at the Emory University School of Nursing. Over the last 10 years, I have been examining the staffing, quality, disparity, and policy in nursing homes using various large administrative data. I am also interested in applying machine learning to aging research and has built various supervised and unsupervised machine learning models. I currently leads a 4-year R01 grant from the National Institute on Aging (R01 AG081282, 2023-2027). I have participated in multiple NIH-funded projects in cancer survivorship previously (R01, U01, UG1, R21, etc.). I was selected into several prestigious aging-related training programs including the RL5 Pepper Scholar from UTMB Pepper OAIC Center, the NIA RCCN Scholars Program in Multidisciplinary Research, the NIA AWARD Network Dementia Workforce Summer Institute, and the R25 Research Methods in Supportive Oncology from Harvard Medical School. My work resulted in 60+ peer-reviewed articles in leading medical and policy journals including The Lancet, JAMA Internal Medicine, JAMA Oncology, JAMA Network Open, Journal of Clinical Oncology, JAGS, JAMDA, Health Affairs, Health Services Research, and Medical Care, as well as two book chapters and 90+ scientific abstracts. I hold national leadership positions at the AcademyHealth, NIA AWARD Network for Dementia Workforce Research, Cancer and Aging Research Group (CARG), and China Health Policy and Management Society (CHPAMS). I serve as a scientific reviewer for the National Institutes of Health, AWARD Network, and CARG, as well as 25+ scientific journals. I have mentored 20+ statisticians, fellows, junior faculty, and students (undergraduate, master, medical, and doctoral). Before coming to Emory, I held faculty positions at the University of Rochester Medical Center and University of Texas Medical Branch. I earned my PhD in Health Services Research and Policy with Data Science training from the University of Rochester.

Areas of Expertise

Publications
Goodwin JS, Xu H. COVID-19 in Nursing Homes-Learning the Hard Way. JAMA Intern Med. 2024 Jul 1;184(7):808-809. doi: 10.1001/jamainternmed.2024.1087.
Bowblis JR, Brunt CS, Xu H, Applebaum R, Grabowski DC. Nursing Homes Increasingly Rely On Staffing Agencies For Direct Care Nursing. Health Aff (Millwood). 2024 Mar;43(3):327-335. doi: 10.1377/hlthaff.2023.01101.
Xu H, Bowblis JR, Becerra AZ, Intrator O. Developing a Machine Learning Risk-adjustment Method for Hospitalizations and Emergency Department Visits of Nursing Home Residents With Dementia. Med Care. 2023 Sep 1;61(9):619-626. doi: 10.1097/MLR.0000000000001882.
Xu H, Mohamed M, Flannery M, Peppone L, Ramsdale E, Loh KP, Wells M, Jamieson L, Vogel VG, Hall BA, Mustian K, Mohile S, Culakova E. An Unsupervised Machine Learning Approach to Evaluating the Association of Symptom Clusters With Adverse Outcomes Among Older Adults With Advanced Cancer: A Secondary Analysis of a Randomized Clinical Trial. JAMA Netw Open. 2023 Mar 1;6(3):e234198. doi: 10.1001/jamanetworkopen.2023.4198.
Xu H, Intrator O, Culakova E, Bowblis JR. Changing landscape of nursing homes serving residents with dementia and mental illnesses. Health Serv Res. 2022 Jun;57(3):505-514. doi: 10.1111/1475-6773.13908.
Mohile SG, Mohamed MR, Xu H, Culakova E, Loh KP, Magnuson A, Flannery MA, Obrecht S, Gilmore N, Ramsdale E, Dunne RF, Wildes T, Plumb S, Patil A, Wells M, Lowenstein L, Janelsins M, Mustian K, Hopkins JO, Berenberg J, Anthony N, Dale W. Evaluation of geriatric assessment and management on the toxic effects of cancer treatment (GAP70+): a cluster-randomised study. Lancet. 2021 Nov 20;398(10314):1894-1904. doi: 10.1016/S0140-6736(21)01789-X.
Xu H, Bowblis JR, Caprio TV, Li Y, Intrator O. Rural-Urban Differences in Nursing Home Risk-adjusted Rates of Emergency Department Visits: A Decomposition Analysis. Med Care. 2021 Jan;59(1):38-45. doi: 10.1097/MLR.0000000000001451.
Xu H, Bowblis JR, Li Y, Caprio TV, Intrator O. Medicaid Nursing Home Policies and Risk-Adjusted Rates of Emergency Department Visits: Does Rural Location Matter?. J Am Med Dir Assoc. 2020 Oct;21(10):1497-1503. doi: 10.1016/j.jamda.2020.04.027.
Xu H, Intrator O, Bowblis JR. Shortages of Staff in Nursing Homes During the COVID-19 Pandemic: What are the Driving Factors?. J Am Med Dir Assoc. 2020 Oct;21(10):1371-1377. doi: 10.1016/j.jamda.2020.08.002.

Teaching
I have extensive experience teaching both health policy and advanced methodology courses to doctoral, master, medical, and undergraduate students.

Research
Over the last10 years, I have been examining the quality, staffing, disparity, and policy in nursing homes using various large administrative and survey data, including national Medicare claims, Minimum Data Set 3.0, CASPER, Care Compare, Payroll-Based Journal data, and LTCfocus.org. In my previous work, I constructed and validated risk-adjusted emergency department (ED) visit rates of long-stay residents, then examined rural-urban differences in rates of ED visits and hospitalizations using Blinder-Oaxaca decomposition methods. I also evaluated how Medicaid policies (payment rates and bed-hold policy) affect ED rates and successful discharge from nursing homes. Since the COVID-19 pandemic, I have focused on the impact of the COVID-19 pandemic on nursing homes and their residents. My 2020 JAMDA article was the first to examine the staff shortages in nursing homes during the early pandemic. A follow-up study found that staff shortages were associated with worse health outcomes among long-stay residents. My recent R01 award from the National Institute on Aging (R01 AG081282) aims to examine the effectiveness and consequences of bans on outside visitors to nursing homes during the COVID-19 pandemic, using a convergent parallel mixed methods design.
As a data scientist, I have conducted several novel studies applying data science to dementia and cancer research. Using national Medicare claims and Minimum Data Set data, I developed a machine learning risk-adjustment method for hospitalizations and emergency department visits of residents with dementia. I found that machine learning can be used in the risk adjustment method. I also implemented an unsupervised machine learning method (K-means) to cluster the Patient Reported Outcomes- Common Terminology Criteria for Adverse Events (PRO-CTCAE) for older adults with advanced cancer. This JAMA Network Open study received media attention from NCI, Medscape, and others. Using the same K-means algorithm, I also reported the changing landscape of nursing homes serving residents with dementia and mental illnesses.

Awards
Summer Training Institute Awardee, NIA Advancing Workforce Analysis and Research for Dementia (AWARD) Network
RCCN Scholars Program in Multidisciplinary Research, NIA Research Centers Collaborative Network (RCCN)
RL5 Pepper Scholar, National Institute on Aging
Early Career Faculty Scholarship Awardee, NIA Pepper OAIC Coordinating Center
Best Poster Award, Denver Post-Acute Care Conference
Student Volunteer Award, AcademyHealth LTSS Interest Group
Dean’s Fellowship, University of Rochester